Untitled 1
Published Research
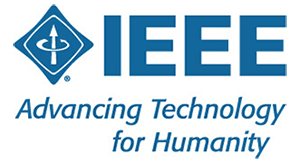
Constructing and Analyzing Criminal Networks - 2014
Abstract:
Analysis of criminal social graph structures can enable us to gain valuable insights into how these communities are organized. Such as, how large scale and centralized these criminal communities are currently? While these types of analysis have been completed in the past, we wanted to explore how to construct a large scale social graph from a smaller set of leaked data that included only the criminal's email addresses. We begin our analysis by constructing a 43 thousand node social graph from one thousand publicly leaked criminals' email addresses. This is done by locating Facebook profiles that are linked to these same email addresses and scraping the public social graph from these profiles. We then perform a large scale analysis of this social graph to identify profiles of high rank criminals, criminal organizations and large scale communities of criminals. Finally, we perform a manual analysis of these profiles that results in the identification of many criminally focused public groups on Facebook. This analysis demonstrates the amount of information that can be gathered by using limited data leaks.
Rade More
ICKD
Detect Abusive Account in Social Network with Arabic Tweets - 2015
Abstract:
Twitter is one of the most popular sources for
disseminating news and propaganda in the Arab region.
Spammers are now creating abusive accounts to distribute
adult content in Arabic tweets, which is prohibited by Arabic
norms and cultures. Arab governments are facing a massive
challenge to detect these accounts. This paper evaluates
different machine learning algorithms for detecting abusive
accounts with Arabic tweets, using Naïve Bayes (NB), Support
Vector Machine (SVM), and Decision Tree (J48) classifiers. We
are not aware of another existing data set of abusive accounts
with Arabic tweets, and this is the first study to investigate this
issue. The data set for this analysis was collected based on the
top five Arabic swearing words. The results show that the Naïve
Bayes (NB) classifier with 10 tweets and 100 features has the
best performance with 90% accuracy rate.
Rade More
IJCSIS
Evaluating Classifiers in Detecting 419 Scams in Bilingual Cybercriminal - 2015
Abstract:
Incidents of organized cybercrime are rising because of criminals are reaping high financial rewards while incurring low costs to commit crime. As the digital landscape broadens to accommodate more internet-enabled devices and technologies like social media, more cybercriminals who are not native English speakers are invading cyberspace to cash in on quick exploits. In this paper we evaluate the performance of three machine learning classifiers in detecting 419 scams in a bilingual Nigerian cybercriminal community. We use three popular classifiers in text processing namely: Na\"ive Bayes, k-nearest neighbors (IBK) and Support Vector Machines (SVM). The preliminary results on a real world dataset reveal the SVM significantly outperforms Na\"ive Bayes and IBK at 95% confidence level.
Rade More
IJCSIS
Improved Micro-Blog Classification for Detecting Abusive Accounts - 2016
Abstract:
The increased use of social media in Arab regions has attracted spammers seeking new victims. Spammers
use accounts on Twitter to distribute adult content in Arabic-language tweets, yet this content is prohibited
in these countries due to Arabic cultural norms. These spammers succeed in sending targeted spam by
exploiting vulnerabilities in content-filtering and internet censorship systems, primarily by using misspelled
words to bypass content filters. In this paper we propose an Arabic word correction method to address this
vulnerability. Using our approach, we achieve a predictive accuracy of 96.5% for detecting abusive
accounts with Arabic tweets.
Rade More
ICCD
A Statistical Learning Approach to Detect Abusive Twitter Accounts - 2017
Abstract:
The increased use of social media has motivated spammers to post their malicious activities on social network sites. Some of these spammers use adult content to further the distribution of their malicious activities. Moreover, the extensive number of users posting adult content in social media degrades the experience for other users for whom the adult content is not desired or appropriate. In this paper, we aim to detect abusive accounts that post adult content using Arabic language to target Arab speakers. There is limited natural language processing (NLP) resources for the Arabic language, and to the best of our knowledge no research has been done to detect adult accounts with Arabic language in social media. We used a statistical learning approach to analyze Twitter content to detect abusive accounts that use obscenity, profanity, slang, and swearing words in Arabic text format. Our approach achieved a predictive accuracy of 96% and overcomes imitations of the bag-of-word (BOW) approach.
Rade More
|
Last Update
7/28/2019 3:24:55 PM
|
|
|